Research
Research Policy
The recent development of measurement and data processing technologies has had a tremendous impact on the medical and healthcare fields, resulting in the accumulation of large-scale and diverse medical big data, including genomic and other omics data and real-world data such as receipts and electronic medical records. While the amount of medical big data is increasing, there is a need to develop new knowledge and new treatment methods that make use of this data.
The “Public Health Informatics Unit” is analyzing medical big data from various perspectives using data science, biostatistics, bioinformatics, epidemiology, and public health to 1) search for risk factors that lead to disease onset and severity, 2) develop algorithms to predict disease onset and severity, and 3) develop new treatment methods and new knowledge using the data. Based on these, we aim to advance the fields of medicine and health science by providing optimal preventive measures and interventions.
Through our research activities, we are also training data scientists in the fields of medicine and health science who can handle big data analysis.
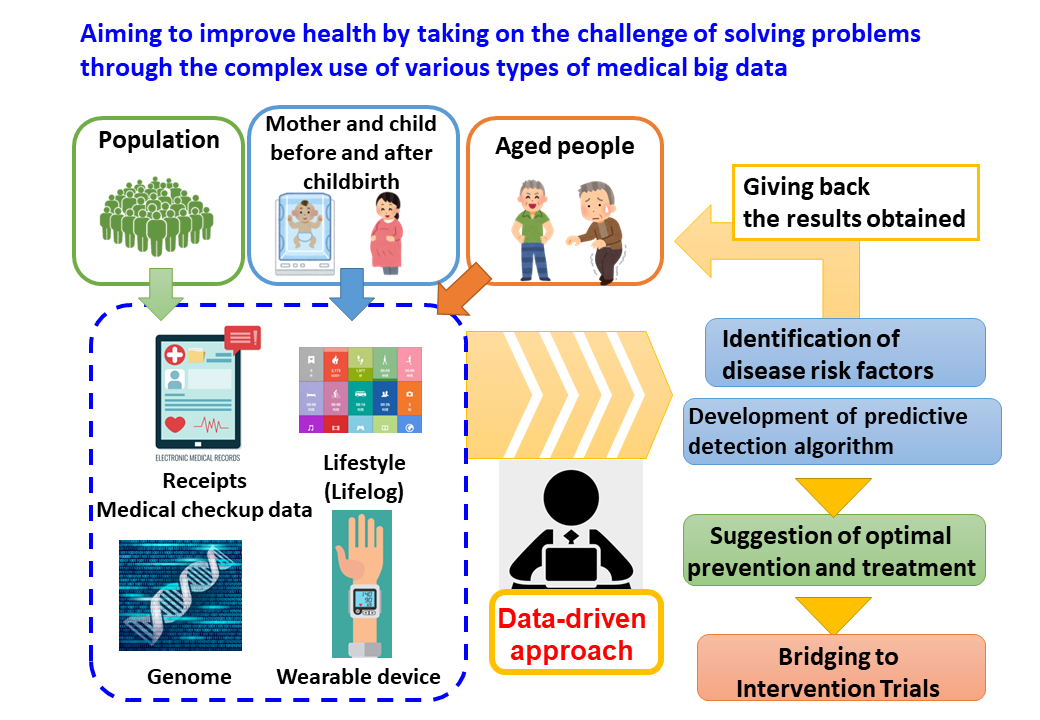
Research Summary
The “Public Health Informatics Unit” conducts research utilizing large-scale medical and health data (Big Data), including genome information, receipts and electronic medical records, to search for risk factors leading to disease onset and severity, and to develop prediction algorithms for disease onset and severity.
Specific research themes include the following medical and health big data analysis research.
- Genomic data analysis of diseases and constitutions
- Development of disease diagnostic and disease onset prediction models utilizing multi-omics data obtained from blood
- Development of a prediction system for the onset of lifestyle-related diseases and mental disorders using big data from health checkups
- Prognosis prediction for newborns using maternal and neonatal data
- Exploration of risk factors that lead to certification of need for long-term care using health checkup data
- Other medical/health science projects
1. Analysis of genomic data on diseases and constitutions
Some people are born taller or shorter than others, some are stronger or weaker drinkers, some are more susceptible to disease than others, and there are individual differences in human constitutions. Genetic factors are thought to be the cause of these individual differences. In cooperation with research institutions in Japan and abroad, our laboratory is exploring genetic factors using genomic data such as single nucleotide polymorphisms (SNPs). So far, we have identified SNPs that contribute to the development of lifestyle-related diseases such as obesity, hypertension, and diabetes, as well as pancreatic cancer and other cancers. We are also developing models for predicting the risk of disease onset and disease progression using this genetic information, with the aim of realizing tailor-made medicine based on genome information.
<Examples of publications>
Nakatochi M, Kushima I et al. Copy number variations in RNF216 and postsynaptic membrane-associated genes are associated with bipolar disorder: a case-control study in the Japanese population. Psychiatry and Clinical Neurosciences 79(1): 12-20 (2025), DOI:10.1111/pcn.13752, Link, Press release
Koyanagi Y, Nakatochi M et al. Genetic architecture of alcohol consumption identified by a genotype-stratified GWAS, and impact on esophageal cancer risk in Japanese. Science Advances 10(4):eade2780 (2024), DOI:10.1126/sciadv.ade2780, Link, Press release
Lin Y, Nakatochi M, Hosono Y, Ito H et al. Genome-wide association meta-analysis identifies GP2 gene risk variants for pancreatic cancer. Nature Communications 11: 3175 (2020), DOI:10.1038/s41467-020-16711-w, Link
Nakatochi M et al. Genome-wide meta-analysis identifies multiple novel loci associated with serum uric acid levels in Japanese individuals. Communications Biology 2:115 (2019), DOI: 10.1038/s42003-019-0339-0, Link
2. Development of disease diagnostic and disease onset prediction models utilizing multi-omics data obtained from blood
Recent developments in measurement technology have made it possible to obtain a comprehensive range of biological information. Such data are called omics data. In our laboratory, we are working to identify factors that enable early diagnosis and prediction of the onset of lifestyle-related diseases and amyotrophic lateral sclerosis, and to develop prediction models, by obtaining various omics data from the blood of local residents.
①DNA methylation(Epigenome)、②miRNA(Transcriptome)、③Protein(Proteome)
<Examples of publications>
Nakatochi M et al. Epigenome-wide association of myocardial infarction with DNA methylation sites at loci related to cardiovascular disease. Clinical Epigenetics 9:54 (2017), DOI: 10.1186/s13148-017-0353-3, Link
Takemoto Y et al. Comparing preprocessing strategies for 3D-Gene microarray data of extracellular vesicle-derived miRNAs. BMC Bioinformatics 25: 211 (2024), DOI: 10.1186/s12859-024-05840-4, Link
3. Development of a prediction system for the onset of lifestyle-related diseases and mental disorders using big data from health checkups
It is considered desirable to intervene appropriately in the development of lifestyle-related and psychiatric diseases from the stage when signs of disease onset appear, rather than starting treatment after the onset of the disease. Therefore, we are searching for risk factors for the onset of these diseases and developing a prediction model to detect signs of disease onset, using health examination data and genome data accumulated daily for residents in the Iwaki area of Hirosaki City, Aomori Prefecture.
Project website(Link):https://coi.hirosaki-u.ac.jp/
<Examples of publications>
Kinoshita F et al. Lifestyle parameters of Japanese agricultural and non-agricultural workers aged 60 years or older and less than 60 years: A cross-sectional observational study. PLoS ONE 18(10): e0290662 (2023), DOI: 10.1371/journal.pone.0290662, Link
4. Prognosis prediction for newborns using maternal and neonatal data
Active management of preterm infants has been shown to improve mortality rates. To this end, accurate prenatal prediction of mortality risk of preterm infants and identification of high-risk individuals is essential for early neonatal care. based on data from over 30,000 Japanese newborns and their mothers, we are developing algorithms to identify high-risk preterm infants using machine learning and AI.
5. Exploration of risk factors that lead to certification of need for care using certification data
In Japan, a long-term care insurance system was introduced in 2000 to provide benefits for the cost of long-term care services to those in need of care. Japan has a markedly aging population, and the number of people certified under these systems is increasing every year. The increase in the number of people certified as requiring support or nursing care causes various problems, such as financial pressure, increases in nursing care insurance premiums, and a rise in the ratio of admissions to nursing care facilities. Therefore, there is a need to extend healthy life expectancy through preventive nursing care interventions. To this end, it is important to provide appropriate interventions before people are certified as requiring long-term care, and it is necessary to identify those at high risk of needing long-term care at an early stage. Our laboratory is developing a prediction model for high-risk individuals by using statistical analysis techniques, machine learning, and AI to search for risk factors for the certification of persons requiring long-term care, based on information on the certification of persons requiring long-term care and health checkup data received from several municipalities in Aichi Prefecture.
Project website(Link):https://c-rex.thers.ac.jp/?page_id=35
<Examples of publications>
Nakatochi M et al. U-shaped Link of Health Checkup Data and Need for Care using a Time-dependent Cox Regression Model with a Restricted Cubic Spline. Scientific Reports 13: 7537 (2023), DOI: 10.1038/s41598-023-33865-x, Link, Press release
6. Others
We also encourage medical/health science assignments that students would like to do themselves with the big data that is currently available to the public or that they can acquire themselves. For example, graduate students have conducted big data analysis research using the Japanese Intensive Care Unit Patient Database (JIPAD), which is run by the Japanese Society of Intensive Care Medicine. Based on the big data in JIPAD, he is estimating the incidence of reintubation after surgery and identifying risk markers. We will continue to train motivated health science students as health data scientists.
<Examples of publications>
Masaki H, Nakatochi M et al. Risk markers for postoperative reintubation of intensive care unit patients: A retrospective multicentre study of the National Intensive Care Registry. Intensive and Critical Care Nursing 87: 103956 (2025), DOI:10.1126/sciadv.ade2780, Link, Press release